When Privacy Meets Performance: How Oblix Helps Enterprises Make the Smart Choice for LLM Workloads
LLMs are quickly becoming a core part of how enterprises operate ā powering internal copilots, knowledge assistants, and productivity tools that help employees work faster and smarter.
But for any organization working with sensitive data, there's a constant tug-of-war between two forces:
š Privacy
"I don't want this prompt ā or this data ā to leave my network."
ā” Performance
"I need the best response, fast ā even if it means calling a powerful cloud model."
So, how do you balance both?
Do you build two different apps ā one for private data, and one for everything else? Do you rely on a single fallback logic and hope it works?
You shouldn't have to choose. Oblix exists to help you do both.
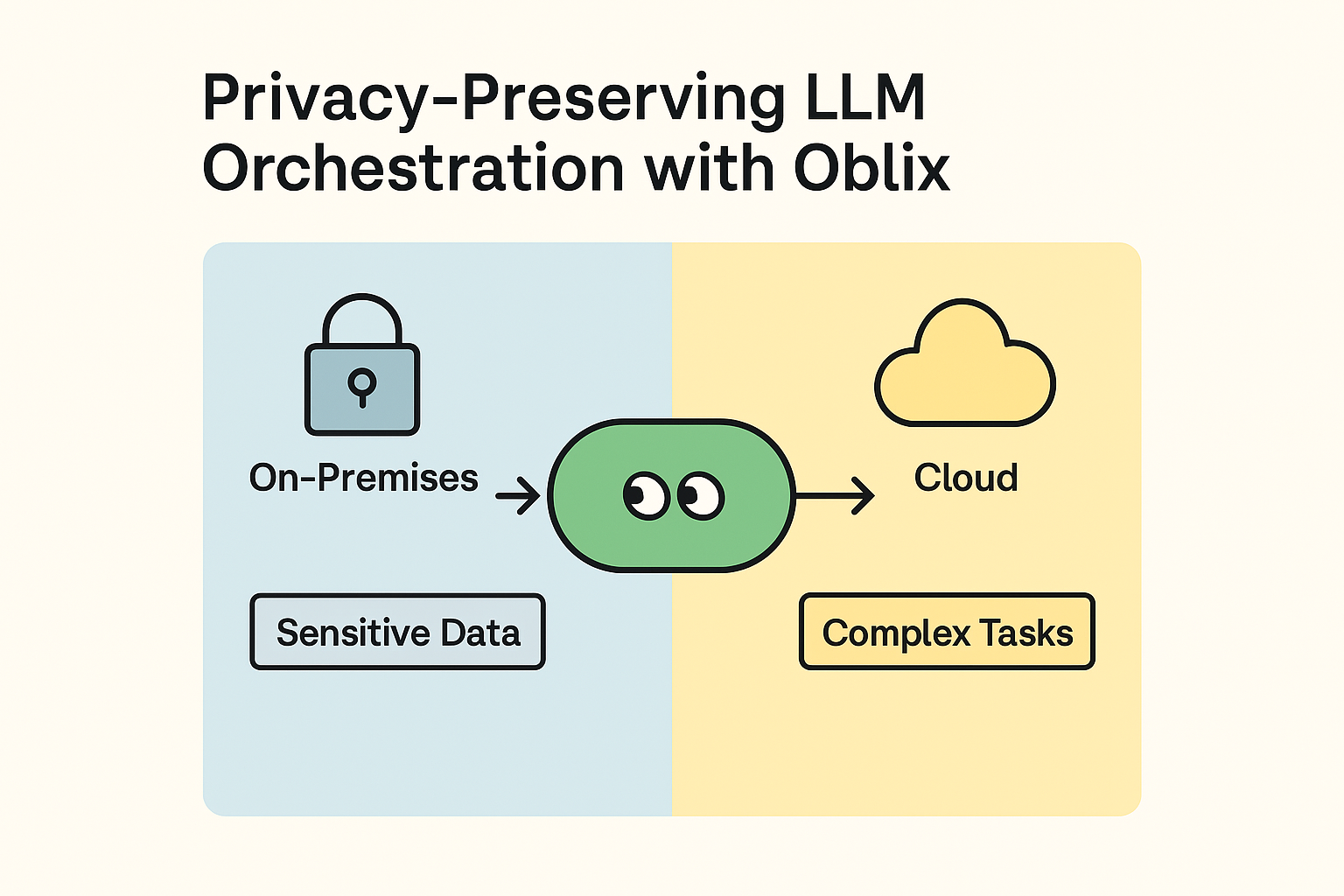
š§ Use Case: Enterprise Knowledge Assistant with Mixed-Sensitivity Data
Let's say your company is deploying an internal LLM-based assistant for employees ā something that helps with:
- Navigating internal docs
- Generating reports
- Summarizing product updates
- Answering company-specific FAQs
- Creating draft content using CRM or sales data
Some of this is generic. Some of it is highly confidential.
Here's where problems arise:
- You want fast, high-quality completions, so you use GPT-4 or Claude.
- But you also need to comply with data handling policies ā certain data must not leave your secure network.
- Even worse, your current system doesn't differentiate between types of queries ā it just pipes everything to the cloud.
š§© The Solution: Oblix + Smart Privacy-Aware Routing
Oblix gives your AI assistant the ability to route prompts intelligently, based on context ā not hardcoded logic.
You define policies:
- If the prompt involves PII, contracts, sales pipelines, or customer info ā run on a local model (e.g. LLaMA 3 on-prem, Ollama, vLLM)
- If the prompt is public-facing, marketing content, general research ā use cloud APIs (e.g. OpenAI, Anthropic)
And Oblix makes the decision in real time, using lightweight on-device agents that evaluate:
- Prompt metadata
- Privacy flags
- Connectivity and latency
- Local model availability
You're not switching between two environments ā you're operating one hybrid LLM system with control and flexibility.
š Example Workflow
Employee A asks: "Summarize this internal strategy doc for our board deck." š Oblix routes locally to your in-house Mistral model on secure infra.
Employee B asks: "Can you draft a blog post on AI trends in retail?" ā” Oblix routes to Claude for richer generation quality and language fluency.
All invisible to the end-user. All governed by policy. All optimized for what matters: either privacy or performance.
š”ļø Why This Matters for Enterprises
Without Oblix:
- You either route everything to the cloud and risk exposure
- Or lock everything down locally and accept slower or less capable outputs
With Oblix:
- ā You meet your data compliance requirements
- ā You deliver high-quality, fast responses where it makes sense
- ā You give your team a seamless experience ā without compromise
š§ Ready to Add Smart Privacy & Performance Logic to Your AI Stack?
We support:
- Local models (Ollama, vLLM, HuggingFace, on-prem clusters)
- Cloud APIs (OpenAI, Anthropic, Cohere)
- Real-time agents that evaluate context + enforce routing logic
We're currently working with teams building internal copilots and secure AI agents. If you're exploring LLM use in sensitive workflows ā let's talk.
š Learn more
Let your AI know when to go local and when to go big.
Join Our Community!
Have questions about implementing a hybrid LLM strategy in your organization? Want to connect with other developers using Oblix? Join our thriving Discord community where you can get help, share your projects, and collaborate with the Oblix team.
Join the Oblix Discord server ā
Enterprise Security First
Oblix is built with enterprise security requirements at its core. Our platform allows you to maintain complete control over sensitive data while still leveraging the power of advanced AI models when appropriate.